Probability is a number
between 0 (never occur) and 1 (always occur) which represents how likely
an event is to occur. Probability is normally defined in terms of the
relative frequency of occurrence of an event from many, many trials.
Probability distributions
or probability density functions (for example, Binomial, Normal,
Lognormal, Gamma, etc.) describe the relative frequency of occurrence
of data values when sampled from a population. There are continuous and
discrete distributions (like random variables). Probability calculations
for discrete distributions involve summation over the discrete variables.
An example of a discrete distribution is:
Binomial |
used to describe
the number of desirable outcomes (successes) in several repetitive
and independent trials, when each trial results in either a success
or a failure only. When the trial can have more than two outcomes,
the multinomial distribution is used. |
Probability density functions
(PDFs) for continuous random variables are:
positive mathematical
functions for all values of the random variable whose total integral
is equal to 1, and where the |
probability of the random
variable to be less than or equal to a certain value a
is equal to the integral of the PDF for values of the variable from
negative infinity to a. |
probability of the random
variable to be between a and b equal to
the integral of the PDF for values of the variable from a
to b. |
probability of the random
variable to be greater than (to exceed) a certain value a
equal to the integral of the PDF for values of the variable from a
to positive infinity (Probability of exceedance). |
The PDF for a continuous random
variables can be thought of as the histogram of relative frequencies plotted
from an infinite number of observations with infinitesimally small intervals.
Examples of continuous probability
distributions:
Normal
or Gaussian |
Two
parameter (mean and variance) symmetrical bell shape distribution.
Probability of the variable to be within 1 standard deviation from
its mean is 67%, within 2 standard deviation 95%, and within 3 standard
deviations 99% |
Log-normal
|
Two parameter
(mean and variance in log units) symmetric distribution of log transformed
values of a random variable |
Gamma
|
Two parameter
(shape and scale) asymmetric distribution |
Figure 1 shows examples of
the fit of gamma and normal distributions to precipitation data whose
distribution is asymmetric. Gamma distribution is a better fit to the
precipitation histogram than the Normal distribution.
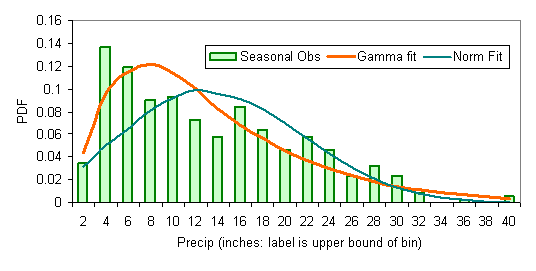 |
Figure 1. Fit of Gamma
and Normal Distributions to seasonal precipitation data at Olympia
Airport, Washington. Mean = 12.5, standard deviation = 8.3, shape
parameter = 1.97, scale parameter = 6.35 estimated from the seasonal
data. |
REFERENCE:
Wilks, D.S., 1995. Statistical
Methods in the Atmospheric Sciences. Academic Press. Pages 64 -93
|